Distributed Learning with a Local Touch: Improving Efficiency in Multiparty Learning
Produktnummer:
189cac94fe1edd4922b9879a63f9be401c
Autor: | Shiva |
---|---|
Themengebiete: | Collaborative AI Collaborative Learning Communication Efficiency Decentralized AI Differential Privacy Edge Computing Education Federated Learning Local Adaptation Scalability |
Veröffentlichungsdatum: | 08.06.2024 |
EAN: | 9783384254221 |
Sprache: | Englisch |
Seitenzahl: | 84 |
Produktart: | Kartoniert / Broschiert |
Verlag: | tredition |
Produktinformationen "Distributed Learning with a Local Touch: Improving Efficiency in Multiparty Learning"
Multiparty learning as an emerging topic, many of the related frameworks and ap-plications are proposed. In this section, we explore the extent of these frameworks and technologies. Yang et al.72 provide a comprehensive survey of existing works on a secure fed-erated learning framework. Bonawitz et al.8 build a scalable production system for Federated Learning in the domain of mobile devices. Konecn`yetal.30 propose ways to reduce communication costs in federated learning. Nishio and Yonetani44 propose a new Federated Learning protocol, FedCS, which can actively manage computing workers based on their resource conditions. Zhao et al.75 notice that conventional federated learning fails on learning non-IID data and propose a strategy to improve training on non-IID data by creating a small subset of data which is globally shared between all the edge devices. Smith et al.63 propose fed-erated multi-task learning, which is a novel systems-aware optimization method, MOCHA.
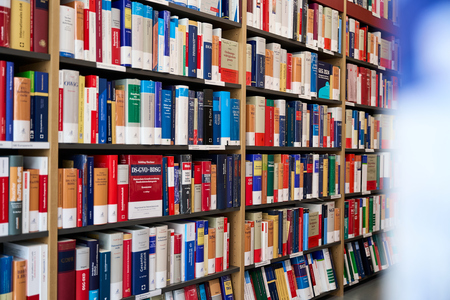
Sie möchten lieber vor Ort einkaufen?
Sie haben Fragen zu diesem oder anderen Produkten oder möchten einfach gerne analog im Laden stöbern? Wir sind gerne für Sie da und beraten Sie auch telefonisch.
Juristische Fachbuchhandlung
Georg Blendl
Parcellistraße 5 (Maxburg)
8033 München
Montag - Freitag: 8:15 -18 Uhr
Samstags geschlossen