Adaptive Online Energy Management Controls for Fuel Cell and Battery Hybrid Vehicles
Produktnummer:
18d16e63abf0e941569473fa44b9ffe6b2
Autor: | Deng, Kai |
---|---|
Themengebiete: | deep reinforcement learning energy management hydrogen powertrain model predictive control |
Veröffentlichungsdatum: | 04.09.2023 |
EAN: | 9783844092042 |
Auflage: | 1 |
Sprache: | Englisch |
Seitenzahl: | 158 |
Produktart: | Kartoniert / Broschiert |
Verlag: | Shaker |
Produktinformationen "Adaptive Online Energy Management Controls for Fuel Cell and Battery Hybrid Vehicles"
Hydrogen energy is a promising future energy source, especially in transportation for zero-pollution improvements. Hybrid systems using hydrogen fuel cells and batteries are crucial for electrification in transportation. These systems offer longer driving range, higher power, and faster charging, making them environmentally friendly and reliable for heavy-duty transport. However, managing multiple energy sources poses challenges. The Energy Management Strategy (EMS) plays a vital role in hybrid powertrains as it controls various components. Objectives include reducing fuel consumption, efficient driving, and component aging. For fuel cell and battery hybrid electric railway vehicles, EMS must minimize hydrogen consumption, sustain battery charge, and leverage route information for energy-efficient driving and fuel cell longevity. This work proposes two strategies to optimize the fuel cell hybrid system. First, a hierarchical EMS based on model predictive control (MPC) uses route information, transforming the problem mathematically and achieving hydrogen reduction, battery charge sustenance, and constraint adherence. Second, a deep reinforcement learning (DRL)-based EMS complements situations with no timely route information. It uses historical data and a fuel cell aging prediction model with DRL method, twin delayed deep deterministic policy gradient (TD3). Simulations of the strategies demonstrate operational cost savings of 14% to 40% compared to conventional methods, meeting design requirements like sustainable battery charging. Hardware-in-the-loop testing validates their real-time capability and effectiveness.
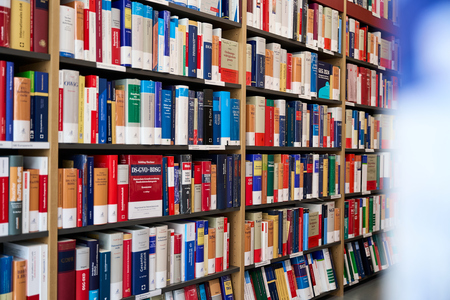
Sie möchten lieber vor Ort einkaufen?
Sie haben Fragen zu diesem oder anderen Produkten oder möchten einfach gerne analog im Laden stöbern? Wir sind gerne für Sie da und beraten Sie auch telefonisch.
Juristische Fachbuchhandlung
Georg Blendl
Parcellistraße 5 (Maxburg)
8033 München
Montag - Freitag: 8:15 -18 Uhr
Samstags geschlossen